What is A/B Testing? The Complete Guide
What is A/B Testing? The Complete Guide
Our ultimate guide on A/B testing, where you'll master the essentials and elevate your skills to pro level. Begin your journey by grasping the fundamentals, and then delve into advanced strategies that will empower you to optimize and enhance your digital experiments with confidence and precision.
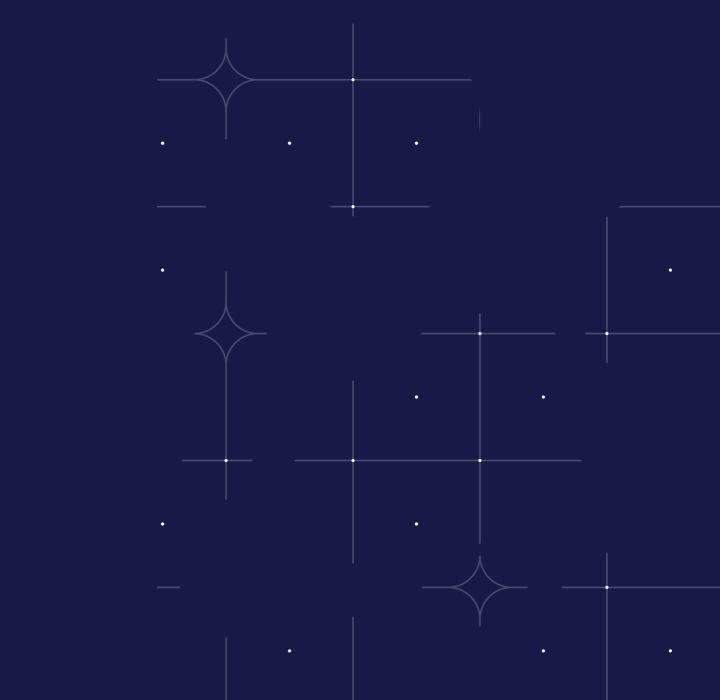
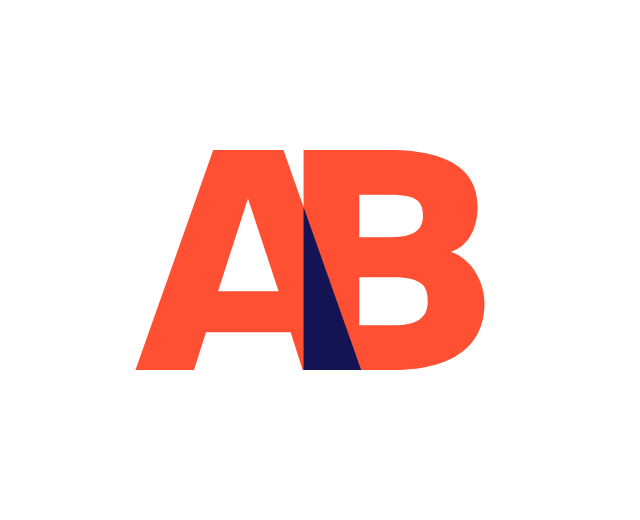
What is A/B testing?Why is A/B testing important?How does A/B testing work?Different types of A/B testingWhen to use A/B testingA/B testing in marketingWhat can I A/B test?Choosing a statistical approachCombining A/B testing with digital experience analyticsGetting started with A/B testingA/B testing toolsBest practices for A/B testingThe impact of A/B testing on SEOCommon mistakesFAQs
A Comprehensive Guide to A/B Testing
Ever wished you could know which elements of your site have the greatest impact? Or understand how people interact with different variations of digital elements on your site? A/B testing can give you hard data to prove these otherwise difficult to answer questions.
The fundamentals of A/B testing are simple to understand, but gaining the most value out of A/B testing tools requires focused and tactful executions. A/B testing allows you to gain fast insights on your website or app users’ behavior and quickly learn where users struggle—while providing the opportunity to improve user experience with speed.
With that in mind, let’s start with the basics of A/B testing.
What is A/B testing?
A/B testing is a common method for determining how your digital elements perform with your users. When running an A/B test, you’re literally testing element A against element B with two different sets of users and comparing the results. Typically, A/B tests are run in digital experience (a website, app or software), where two sets of visitors are delivered the experience with only one distinct element changed.
Through one or multiple A/B tests, web teams test the outcome of each variation, then use the insights to make data-driven decisions for a better user experience—typically with the end goal of driving more conversions.
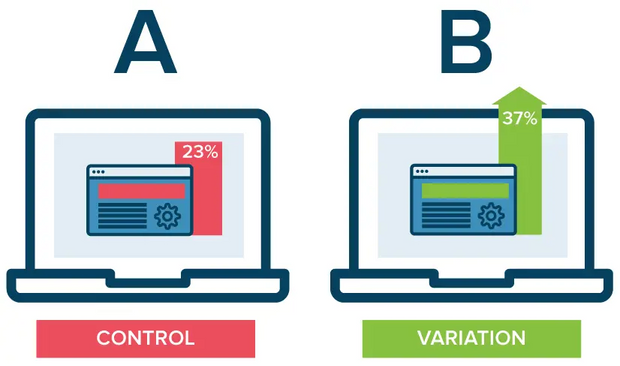
Why is A/B testing important?
A/B testing is a critical tool in achieving user-centric design. By giving you insights into how elements on your site change the user experience, A/B tests can help you optimize that experience and ultimately drive more sales.
Here are four goals A/B testing can help web teams achieve:
Conversation rate optimization (CRO)
Conversion rate optimization is the process of increasing the number of users who take a desired action on your website or mobile app. To do this, you need to know how users are interacting with your site. Through A/B testing the various elements that you want to achieve high engagement, you can validate whether your current elements are working—or test new element ideas to better optimize performance.
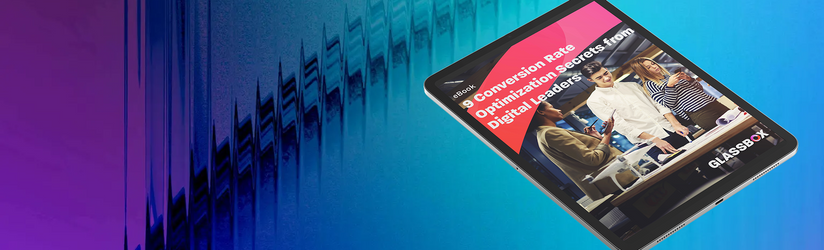
9 Conversion Rate optimization Secrets from Digital Leaders
Get the eBookLower bounce rates
If your bounce rates are high on certain pages of your site—or high overall—A/B testing can help you determine why users aren’t connecting with your content. You can test current elements of your site against ideas that could drive higher engagement and make data-driven decisions that could potentially improve your bounce rates.
Fast insights
A/B testing is an extremely quick way to determine which areas of your site are underperforming. For example, if you’re seeing low engagement with certain pages of your site, running A/B tests on key elements of the page can give you quick feedback on how to alter the page for higher engagement.
Sales growth
The end goal of A/B testing is to increase conversions and ultimately grow sales. If you’re delivering content that users connect with and are able to take action on, sales will follow an upward trajectory. Likewise, you’ll build a loyal customer base that will also fuel sales growth.
How does A/B testing work?
With A/B testing, you are testing two variations of digital elements such as headlines, calls to action (CTAs), content length, button size, button placement, etc. Users aren’t aware of the testing as it runs behind the scenes. Tests are run in real time with two sets of different users. Once the results are in, you compare the data to see which test—A or B—performed the best. A/B testing informs the strategy behind your website or mobile app design and content—empowering you to serve up the elements that engage users the most.
Different types of A/B testing
There are three types of A/B testing—traditional A/B testing, split testing and multivariate testing. Here, we’ll describe each type of test method:
Traditional A/B testing
True A/B testing evaluates the performance of one digital element at a time, such as CTA button or a color. The A group is how the element is currently presented on your website or app and the B group is a change that you want to test.
Split testing
While similar and often used interchangeably with A/B testing, split testing does have one distinguishing feature: the traffic is a 50/50 split between the element being tested. In traditional A/B testing, the traffic can be split any way you want: 70/30, 40/60, etc. But split testing is always an equal split.
Multivariate testing
In multivariate testing, digital teams test multiple elements at the same time. You can test color, font, headlines, images, CTAs or any other digital element simultaneously. The goal with multivariate testing is to find the best performing combination of various digital elements. Note: Since the number of variations is more complex in a multivariate test, these A/B tests require a high amount of traffic during the test to produce statistically significant results.
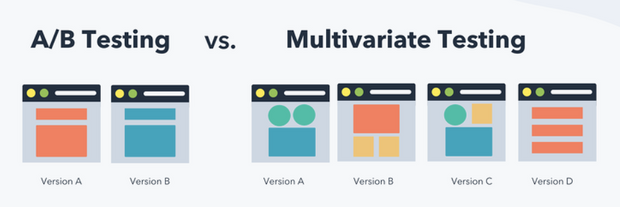
When to use A/B testing
A/B testing can be used at any point to optimize performance for elements on a website or mobile app. You can start with conducting A/B tests on elements you believe are underperforming. Or, A/B testing can be used to validate what you think is working by giving you the data to back up your assertion. As part of an ongoing marketing strategy, it’s wise to continually conduct A/B testing—even on elements that ARE performing well—to see if there are ways to improve.
A/B testing in marketing
Since marketing plays a significant role in website and app development, marketing is one of the principal players in A/B testing. Marketers use A/B testing to improve the performance of digital assets and create a better customer experience. The data derived from A/B testing can also be valuable in demonstrating that marketing efforts are working, which can prompt more investment from the business.
Beyond marketing, A/B testing can also be useful for testing product or service pricing. By varying the pricing on your website or app, you can test the market’s appetite for pricing levels and find the sweet spot that converts users. A/B testing is also frequently used in email campaigns to determine which design elements, content and calls to action have the best performance.
What can I A/B test?
A/B testing can be conducted on a wide variety of your digital elements, such as:
CTAs
Headlines
Offers
Images
Color
Fonts
Content
Form fills
Page length
Devices
Newsletter sign-ups
Once you have the comparison data through A/B testing, you can optimize your website or app to improve the overall performance with users.
Choosing a statistical approach
Now that you’ve conducted A/B testing, it’s time to interpret the test results. There are two statistical approaches commonly used in A/B test data interpretation: Frequentist and Bayesian.
The Frequentist statistical approach
This is the more traditional statistical approach for A/B testing that uses only the data from one experiment. The Frequentist approach starts out with a hypothesis to determine if there is a difference between the A and B groups. For example, if you believe there is no difference, then it is a “null hypothesis.” Otherwise, you hypothesize that there is a difference between the two groups. From there, you test out your hypothesis to see which one is true.
The Bayesian statistical approach
The Bayesian approach is less conventional than the Frequentist approach, but it is growing in use. This approach overlays the data from the current experiment with data from prior similar experiments to draw a conclusion about the probability of a belief. This differs from the Frequentist approach in that it involves assigning a probability to a belief, rather than just relying on the testing results alone.
Benefits of combining A/B testing with digital experience analytics
Measuring A/B testing results should go beyond just looking at conversions. More than examining the beginning and end interactions of your users, you need to explore what happens in the middle of the interaction. By combining A/B testing with digital experience analytics, you get the full picture of the user journey and can make better business decisions.
Digital experience analytics fills the gap in traditional A/B testing—helping you make more insightful, data-driven decisions. The three benefits of taking this approach are:
Analyzing your users’ behavior throughout the journey
A/B testing can help you learn whether users are converting—and which elements help them convert better. Digital experience analytics will reveal why they are or aren’t converting. You will understand the entirety of the digital experience, where users are getting frustrated and what’s causing their struggles. When used in combination with an A/B test, the experience information can help you explain why certain winning elements performed better in an A/B test and use this insight to build elements to test on other pages.
Visualizing exactly where your users are spending their time
With specialized digital analytics tools, you can replay user sessions to see exactly what your users are doing on a specific webpage you’re testing. This can be useful prior to beginning an A/B test, allowing you to pinpoint where users are spending their time. As you map interactions with the existing elements in an experience, playing real user sessions can help you decide which elements have the potential to make the biggest impact when improved. Then, replaying sessions from during the test lets you see how users interacted with the specific elements on the page—and how those interactions differed between A and B.
Uncovering how much revenue is being generated
Attaching A/B testing data to the data around conversions and the revenue generated from specific user actions brings additional value to the test results. With these insights, you can link each A/B test performed with its potential financial impact. This information is especially useful in gaining top-level buy-in for continued investment in digital optimization testing.
Getting started: An A/B testing framework
There are a variety of foundational steps to performing an A/B test and improving conversion rate optimization. The best results take place when A/B tests are performed with meticulous attention to detail and documentation, from research and developing a hypothesis to prioritization, testing and reviewing/deploying results.
Research
The first step to take in performing an A/B test is research. It’s the foundational element of testing and the key to success. To help you understand your audience, you’ll want to use both qualitative and quantitative data. While quantitative data is often easier to understand and measure against (because it uses numbers that can be counted or measured), it often lacks the human element of what makes your users’ needs unique. To gather qualitative data, use interviews, surveys, feedback and other methods to understand a user’s behaviors, motivations and other variables in their website or app experience.
Hypothesis
Once the research has been conducted, it’s time to draw a hypothesis. Use the research data to formulate a hypothesis that gets to the heart of the audience–your users. A good hypothesis for conversion rate optimization should propose a solution to the identified issue and predict the expected results of the solution. It also increases the probability of the right assumption and success.
Prioritize
With solid research and a good hypothesis, it’s time to prioritize your first test. Use the P.I.E framework to rank each element by potential, importance and ease. This framework for CRO projects brings discipline to A/B testing programs and creates a roadmap for future testing.
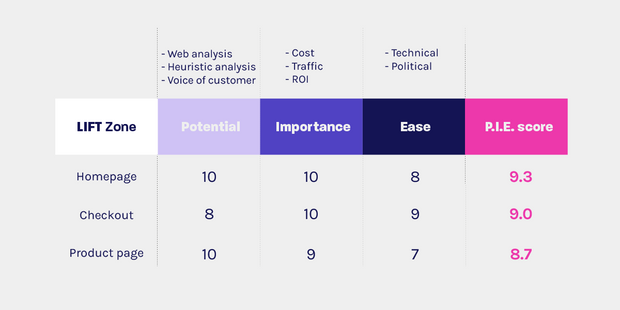
(P) Potential–how much improvement is possible on the page? Use all of the collected and analyzed data along with user scenarios to determine potential.
(I) Importance–how valuable is the traffic to the page? The most important pages have the highest volume and costliest traffic.
(E) Ease–how difficult will it be to implement a test on a page? Take into consideration technical implementation, organizational and political barriers.
Testing
Now, it’s time to determine what type of testing you want to perform–A/B testing, split testing or multivariate testing. Next, consider how much traffic you need (sample size) to get statistically significant results. Tools like a sample size calculator can help you determine the right sample size for your test. The length of the test should be at least 1-2 weeks and no more than 8 weeks, depending on the buying cycle, site traffic volume, shopping patterns and other variables.
Review and deploy Results
The last and crucial step is to analyze your A/B testing results and determine which variation won. If you conducted a simple A/B testing of two variations and there’s a clear winner, you can move to deploy the winning result on the live site. With multivariate testing, you may want to test the original control against the winning variation for further insights.
When testing is finished, it’s time for implementation. You have the UX changes you want to make, so collaborate with your development team to make and prioritize the changes for go live.
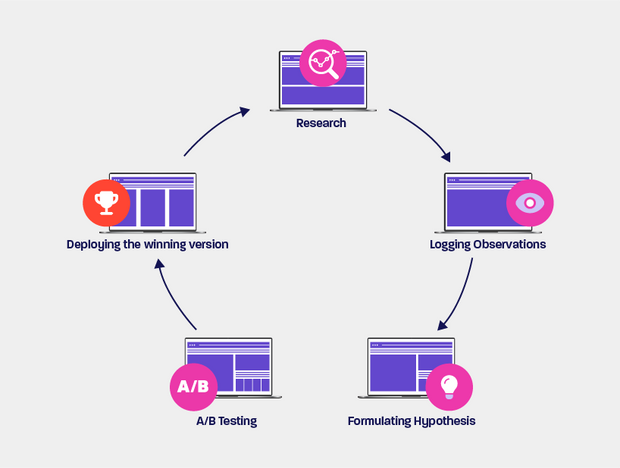
What A/B testing tools do you need to get started?
There are many A/B testing tools available for all types of companies—from small and mid-sized organizations to large enterprises. Depending on the organization’s needs, A/B testing software should accommodate both server-side and client-side testing, split and multivariate testing and code and WYSIWYG editors.
Organizations should also choose an A/B testing software that provides website personalization and feature toggle capabilities as well as digital commerce and web content management features. When combined with a digital experience platform, A/B testing software can unlock even more digital insights and provide a frictionless journey for those performing A/B testing.
Best practices for A/B testing
There are a wide range of best practices for A/B testing. By following these seven best practices, you are better positioned to successfully perform A/B tests.
Test high performing content
Test the pages that users already engage with the most such as your home page, blogs, contact, or other lead generating pages like eBook and webinar sign-up pages. While it may be tempting to test lower performing pages to try to make improvements, the insights you gain will likely have a smaller total impact.
Nail the hypothesis
If your hypothesis is flawed, your results will also likely be flawed. Also, testing without a hypothesis—just to see what you uncover—isn’t a good use of time and effort. A good hypothesis should focus on a one problem that needs to be solved, it should be able to be confirmed or disproven, and it should center around a goal.
Use good data
Make sure you are producing reliable data with enough data points to ensure your results are both statistically accurate and statistically significant. Good data will lead to reliable results that can help you make the right decisions about your content and drive increased user engagement.
Go the distance
Make sure to let the A/B test run its course. Stopping A/B testing early—because you think you already have the insights you need—may deliver inaccurate results.
Be patient
You may not see results right away, but don’t pull the plug on the A/B test. It can take time to drive results so patience is definitely a virtue in A/B testing. If you’ve done the work up front to ensure your test is worthwhile, any result you get will hold value—even if it’s a clue that leads you to test something new.
Go big
Making only slight alterations isn’t likely to drive significant changes in your website or app users’ behavior. Make the A and B content and graphic variations different enough so you can really gain insight into your users’ preferences.
Continually test
A/B testing isn’t a one-and-done exercise. Optimizing your digital experience will require you to dig past the obvious and continue to scrutinize every piece of your users’ journeys. And, since users’ preferences and behavior can be fluid over time, even elements you’ve previously A/B tested should be continually revisited to ensure they’re still producing the best results.
The impact of A/B testing on SEO
Since a significant part of Google’s ranking algorithm focuses on user experience, improving this experience through A/B testing will inevitably produce favorable results for your long term SEO strategy. But what about the short-term impact of conducting the A/B tests themselves?
According to Google, if A/B testing is done right, it won’t impact SEO. However, if done wrong, SEO could be negatively impacted. Below are a few of the risk factors that could hurt your SEO rankings during an A/B test.
Duplicate Content
Google’s Algorithm is trained to penalize websites that publish duplicate content across more than more page. This poses a problem for A/B tests since the technique requires you to publish variations of similar page content. You don’t want to trigger duplicate content warnings from the search engine. If the content you’re testing doesn’t typically see much search engine traffic, having duplicate content won’t impact your SEO in a significant way. However, if the page you’re testing has SEO significance, you’ll want to use a canonical link element, an HTML element that will allow you to tell Google which version of the page should be used as the “primary” page it displays in search results.
Cloaking
Google frowns on “cloaking” and will rank pages lower if this practice is used. Cloaking is a term used when sites present different content externally to the users of the site, than they do to the search engines. It is seen as an attempt to manipulate search rankings and mislead users. Therefore, choosing to hide the A/B testing pages from the search engines is not advisable. You should always present the same content to users and search engines to prevent cloaking.
Slow page loading
A slow loading page directly impacts user experience, so Google’s algorithms will rank that page lower. The same goes for if the entire site is slow to load. Depending on the tool you choose to conduct A/B tests, the potential harm to your page load speed can differ. However, it’s generally true that A/B testing will slow page loading. Taking the steps to ensure your page load speed is optimized in every way possible, such as deploying A/B testing on the server side, will make sure your page speed is minimally impacted.
Using the wrong redirect
Since A/B testing involves sending users from one URL to two different landing locations, it’s important to assign the right redirect code. The temporary 302 redirect should always be assigned with A/B variations to let Google know which page to index and which to not index. Using a 301 redirect will tell Google that the redirect is permanent and all ranking power will be passed to a new URL—this is not what you want.
Common mistakes
There are many ways A/B testing can go wrong. Having a bad hypothesis, stopping the test too soon and choosing the wrong A/B testing tool are some of the major culprits. Let’s take a look at these A/B testing mistakes that can throw your efforts off track.
Having a bad hypothesis
You may think you understand what your users want, but that shouldn’t underpin your hypothesis. The same goes for gut feelings or personal opinions. Taking the time upfront the get to the right hypothesis helps ensure the insights you learn during testing will be valuable.
Stopping the test too soon
If you stop testing at the first sign of a significant insight, your efforts will likely produce invalid results. Let the testing run its course to get complete insights that can drive good digital decision-making.
Selecting the wrong A/B testing tool
There are many A/B testing tools available and it can be an arduous process determining which one is right for your organization. Work with an A/B testing provider to find the tool that will deliver the digital insights you want.
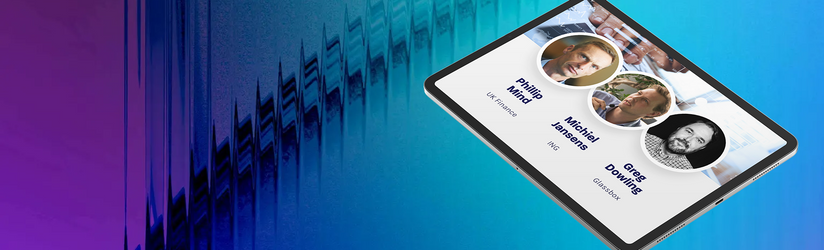
Putting Insights and Experimentation at the Heart of Your Digital CX Strategy
Watch the videoFAQs
Check out the A/B testing FAQs if you’re short on time or are looking for a quick cheat sheet on A/B testing.
What is A/B testing?
A/B testing is a common method for determining how your digital elements perform with your users. It involves testing element A against element B with two different sets of users and comparing the results.
How to split traffic for A/B testing?
If you have two variations that are being tested, you can split the traffic 50/50 or assign a different percentage, like 60/40 or 70/30 (any combination), if you don’t want each variation to be equally weighted. The unequal split is typically chosen when you believe you are testing an inferior variant and don’t want to incur the higher costs typically associated with an equal split. Or, if you’re testing whether to raise pricing, you may only want to show the higher pricing to fewer users.
What is A/B testing in marketing?
Marketers use A/B testing to improve the performance of digital assets and create a better user experience. The data derived from A/B testing can also be valuable in demonstrating which marketing efforts are working—and which are not.