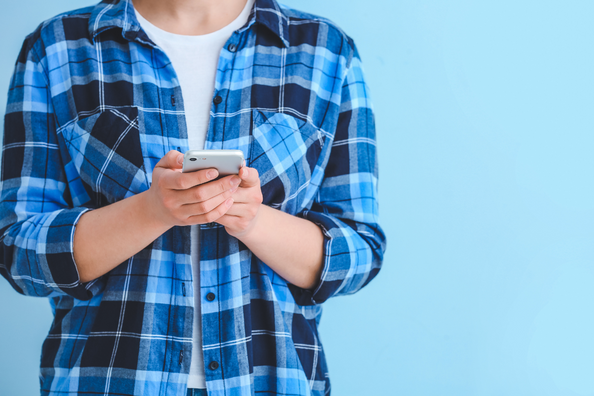
Voice of the Silent: AI is transforming customer feedback before our eyes
Crickets. A gently whirling fan. Tumbleweeds blowing down a desolate country road. These are all currently making a lot more noise than your customers. Most of them, anyway. On average, Voice of the Customer (VoC) data is sourced from just 4% of users — but what if you had the ability to listen to your silent majority for a change? With AI, now you can.
It’s 2014 and the tech industry’s “decade of disruption” is underway. Chatbot behemoth Drift won’t exist for another year. The Internet is going all in on the ice bucket challenge. Less than 1% of companies have the ability to measure customer engagement. Everyone is talking about digital transformation. With AI taking off, Gartner issues a bold proclamation: “Customer experience is the new competitive battlefield.”
Fast-forward to 2024, and the relationship between organizations and their customers is unrecognizable. To keep up with skyrocketing customer demands and continually expanding customer journeys, teams have spent the interim years building out mature CX functions, with robust tech stacks to capture, measure and analyze interactions over a dizzying combination of channels and touch points. Brands’ understanding of customer feedback has also undergone a radical transformation, with Voice of the Customer (VoC) now cemented as its own dedicated science.
By all accounts, everyone should be celebrating a CX equivalent of the renaissance. But teams continually struggle to provide seamless customer experiences at scale:
80% of consumers think customer experiences need to be improved.
70% of CX leaders don’t believe CX efforts across their company have achieved the intended results.
Poor customer experiences cost U.S. businesses $83 billion in marketing each year.
U.S. companies lose $136.8 billion per year from avoidable consumer switching.
The problem is, technology has evolved enough for customers to expect effortless, personalized experiences 24-7—but not so far that organizations can easily or consistently provide them. Because let’s be honest—for all the technological advances, VoC platforms still offer limited insight into a customer’s shifting wants, needs, preferences, priorities, perceptions and struggles, especially during complex buyer’s journeys.
Until now. To forward-thinking CX teams, AI is the strongest power tool in their arsenal—and we’re not talking about chatbots and personalized recommendations.
Thanks to Machine Learning, AI can now translate customer behavior into quantifiable feedback, so you can finally listen to your silent majority—even (and especially) if when they blow off your satisfaction surveys. Here’s how it all works, and why we think AI will trigger the next major wave of disruption in CX.
The biggest VoC limitations
VoC has served us well in the past—it’s the only way to systematically collect and analyze customer experiences for improvement. However, traditional tools and processes often starve teams of meaningful data and lead to lackluster results:
93% of CX leaders report using a survey-based metric (i.e. CSAT or CES scores) as their primary means of measuring CX performance.
Only 15% of CX leaders said they were fully satisfied with how their company was measuring CX.
Just 6% of CX leaders expressed confidence that their measurement system enables both strategic and tactical decision making.
1. Sacrifice insights for ease
VoC surveys can inadvertently compromise the same customer experience they’re trying to measure. This prompts CX teams to strip down feedback requests so they cause as little inconvenience, delay or interruption as possible—users can rate an hour-long live chat with just a single emoji. Even with more “extensive” VoC surveys, you’re still advised to follow general best practices and stick to six questions or less.
While ease and usability are rarely a bad thing, mitigating this friction also means minimizing potentially critical insights—you may win the battle with an effortless VoC survey, but still lose the war when it comes to understanding the overall customer experience.
2. Only reflect a tiny vocal minority
The bigger problem? Even after making it as easy as possible for customers to provide feedback, a tiny minority will actually take you up on the opportunity. On average, VoC data is sourced from just 4-7% of users—in our own research, we’ve found it to be closer to 4%. That leaves 96% whose perceptions are unknown. We like to call them the silent majority or voice of the silent. Don’t forget, 91% of customers who are unhappy with a brand will leave without complaining.
3. Follow the same rules for every user
If it wasn’t hard enough to get customers to provide feedback, CX teams also face limited ability to personalize the timing of these requests. Sure, you can surface an exit-intent popup as users are about to leave your site, or a satisfaction survey after they’ve closed a chat window with a support agent.
However, these rules are typically the same for all users across your site or app.
Timing is everything, but there’s no way to automatically make a request at the opportune moment for an individual customer, when you have the best chance to prompt a response and actually learn what happened. Similarly, it's impossible for traditional feedback requests to respond to specific actions which indicate users are struggling, i.e. dead clicks or rage clicks.
In the end, traditional, survey-based VoC tools often leave CX teams famished for meaningful insights. This isn’t going to cut it for present day customer journeys. Not when:
One in three customers would leave a brand they love after just one bad experience.
80% of B2B buyers will look for another supplier if not offered consistent experience across channels.
78% of customers have backed out of a purchase because of a poor customer experience.
73% of customers consider experience a more important factor in their purchase decisions than price or product quality.
place high importance on previous positive customer experience with a brand when considering a new purchase
89% of consumers have done business with a competitor following a poor customer experience.
Hitting the bulls eye is hard enough without a blind fold on. For most CX teams, the majority of these experiences are still shrouded in mystery—especially when it comes down to contextual insights and nitty gritty details. This makes it almost impossible to routinely deliver what customers are looking for. Now, organizations are turning to other options.
Gathering feedback from your silent majority with AI
You’ve heard all the adages.
Talk is cheap.
Actions speak louder than words.
But when it comes to the voice of the customer, voice is usually the operative word. Whether it's an emoji reaction, survey response or indirect brand mention on Twitter, traditional VoC processes still put the onus on customers to expressly communicate their thoughts, feelings and perceptions.
AI is now taking this model and turning it on its head—by translating user behavior during interactions into quantifiable feedback. It does this in a couple different ways:
Machine learning: Machine learning algorithms process and “learn” from incoming data through pattern recognition, and can then make decisions or predictions without being explicitly programmed to.
Natural Language Processing (NLP): NLP processes text-based human language and then uses machine learning to identify patterns and contextualize data. It then generates metadata—“data about data”—which is sorted into dynamic, self-adapting informational hierarchies or taxonomies.
So what does all this mean for you? Essentially, you can leverage AI to take customer-rated interactions and automatically determine how many comparable sessions occurred across your entire user base. This enables CX teams to:
1. Use survey feedback to find and quantify similar experiences across all users
Let’s say 15% of your NPS survey respondents rated their experience a 6 out of 10. Since only 4% of users typically provide feedback, we know that this 15% is actually closer to 0.6% of your total traffic. Not exactly the most accurate representation.
However, since AI captures, categorizes, recognizes and contextualizes data, it can segment this 0.6% and generate a step-by-step record of actions taken by individual users during their session, leading up to their 6 NPS score. AI can then detect any common data points—patterns, errors, sources of friction—not only among the 0.6%, but all users.
Even though the vast majority didn’t respond to the survey, it’s safe to assume that the same sequence or combination of actions would result in a similar experience—and NPS score. Now you have a way to actually quantify “unrated” sessions that constitute the bulk of your traffic.
2. Identify and prioritize sources of friction that impact the most users
User feedback can be helpful in identifying sources of friction, but isn’t the most reliable way to determine scope of impact. Imagine four customers complained to your chatbot about an unresponsive checkout page. Let’s also say that each person described the error in a completely different way—so traditional VoC tools can’t compare terms.
How can you tell if these are isolated incidents or affecting a larger volume of traffic? With AI trained on actions instead of words, it can form a timeline of user actions across these particular sessions and compare them with all other sessions that occurred over the same period. While only four customers actually complained, four thousand may have encountered dead clicks and page reloads on the same page, indicating they were similarly affected.
This way, you can not only identify and prioritize which errors to correct first, but also more efficiently locate the root cause.
3. Directly see how friction impacts revenue
Let’s go back to our previous example, where four customers reported an error on the checkout page. AI can go further than comparing actions across all sessions to determine how many users were actually affected. It can also quantify the impact on revenue.
Pretend that over the past week, four thousand sessions encountered the same checkout error, causing users to leave without making a purchase. AI can calculate the average order during that time—$150—and determine this single error potentially cost $600,000. If the error continues to affect the same proportion of sessions moving forward, that’s $2.4 million in lost revenue per month or nearly $29 million per year.
In this way, AI adds depth and context to customer experiences by providing a more thorough, direct and accurate connection with revenue.
AI is shaking up VoC on a massive scale.
Organizations have a radically different understanding of the customer journey than they did back in 2014. It’s time to think differently about VoC as well. Meeting or exceeding expectations for every single customer requires an exhaustive supply of accessible, actionable data—and you’ll need a lot more than satisfaction surveys to find it. The teams positioned to gain the biggest competitive advantage aren’t doubling down on “tried and true” methods. Instead, they’re leveraging AI to listen to silent customers through their actions rather than words.